Postdoctoral researcher Yang Zhong from Prof. Li Guohui’s group at HUST has had his paper, “Universal Set Similarity Search via Multi-Task Representation Learning”, accepted as a full paper by ICDE2025—a top-tier global forum for database and data systems research. As one of the three premier conferences in database research, ICDE is rated as A-level Conference by China Computer Federation (CCF).
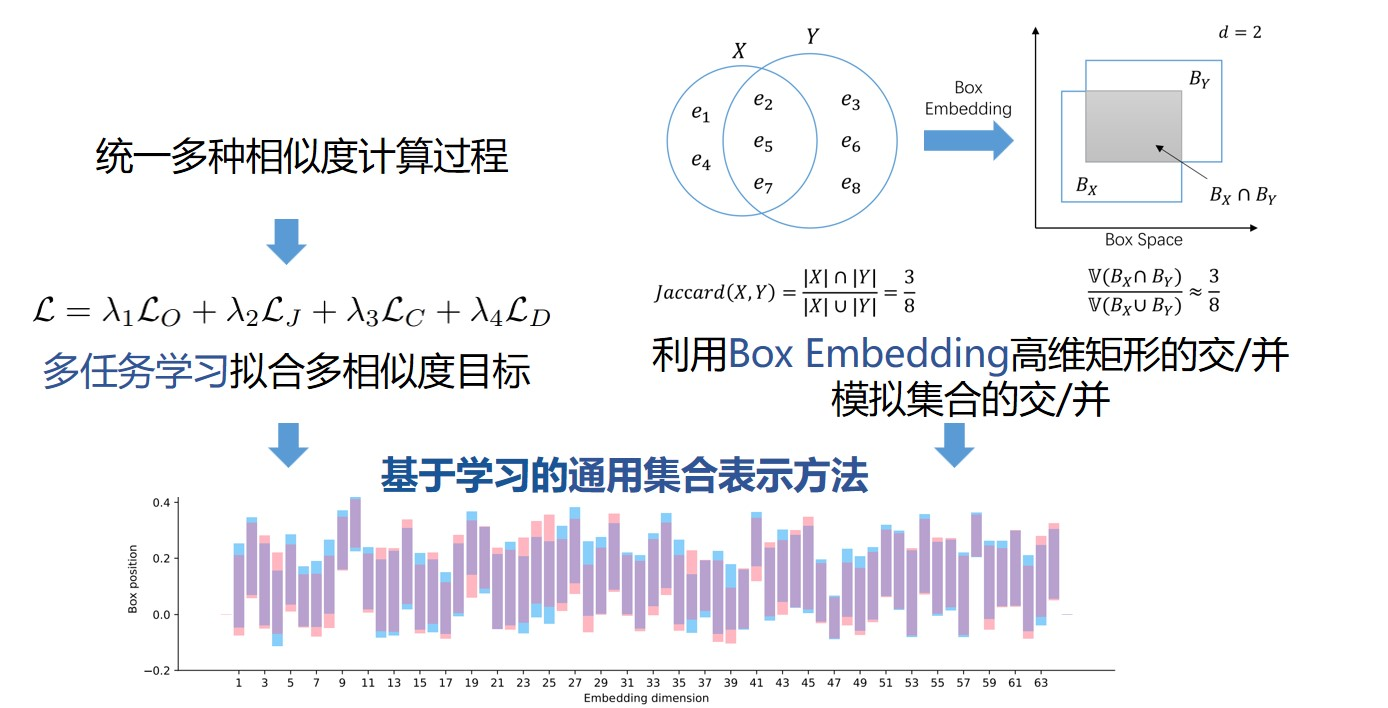
Figure 1: Universal set representation based on Box Embedding
Set similarity search is a basic operation in data processing and is widely applied in data cleaning and integration, information retrieval, plagiarism detection, genetic testing, etc. The most commonly used set similarity measures include Overlap, Jaccard, Cosine, and Dice. However, traditional methods cannot solve the search problem simultaneously under different similarity metrics and query types. This is because they are usually designed for specific similarities and are extended and transformed to adapt to different similarities, which leads to an obvious deviation in the validity of different similarity metrics. To solve this problem, the article firstly explores a multi-task representation learning based on Box Embedding (MTB), as shown in Figure 1.
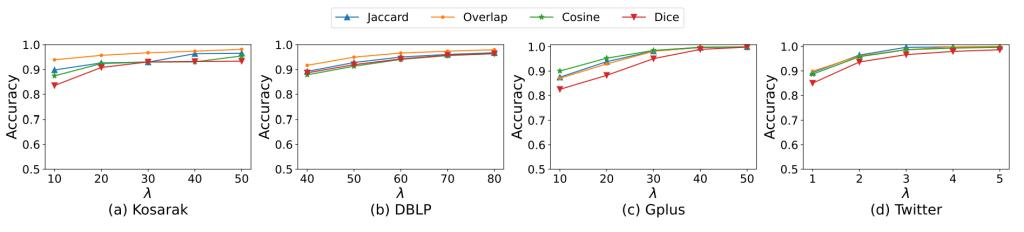
Figure 2: Usearch shows high accuracy on different similarity metrics and datasets
Secondly, it studies the universal set similarity search algorithm (USearch) based on the representation, which is a general search framework that accommodates various set similarity and query types. Experiments reveal that the method shows good generality and effectiveness, especially on large datasets, while ensuring high data query accuracy. Some of the results are shown in Figure 2.