July 29, 2024
In recent days, a total of 9 papers from the research teams of Professor Bai Xiang, Professor Xia Tian, and Associate Professor Tang He from our college have been accepted by the European Conference on Computer Vision (ECCV 2024), showcasing the latest research achievements in the field of computer vision.
ECCV is one of the top three international conferences in the field of computer vision (alongside CVPR and ICCV). This year, the conference received 8,585 valid submissions and accepted 2,395 papers, resulting in an acceptance rate of approximately 27.9%. The conference is held every two years and gathers professionals from both academia and industry. This year, it will take place in Milan, Italy, from September 29thto October 4th.
OPEN: Object-wise Position Embedding for Multi-view 3D Object Detection
Accurate depth information is crucial for enhancing the performance of multi-view 3D object detection. Despite the success of some existing multi-view 3D detectors utilizing pixel-wise depth supervision, they overlook two significant phenomena: 1) the depth supervision obtained from LiDAR points is usually distributed on the surface of the object, which is not so friendly to existing DETR-based 3D detectors due to the lack of the depth of 3D object center; 2) for distant objects, fine-grained depth estimation of the whole object is more challenging. Therefore, we argue that the object-wise depth (or 3D center of the object) is essential for accurate detection. In this paper, we propose a new multi-view 3D object detector named OPEN, whose main idea is to effectively inject object-wise depth information into the network through our proposed object-wise position embedding. Then, we utilize the proposed object-wise position embedding to encode the object-wise depth information into the transformer decoder, thereby producing 3D object-aware features for final detection. Extensive experiments verify the effectiveness of our proposed method. Furthermore, OPEN achieves a new state-of-the-art performance on the nuScenes test benchmark.
Authors:Jinghua Hou,Tong Wang,Xiaoqing Ye,Zhe Liu,Shi Gong, Xiao Tan,Errui Ding,Jingdong Wang,Xiang Bai
Affiliation:Huazhong University of Science and Technology,Baidu Inc.
Seed: A Simple and Effective 3D DETR in Point Clouds
Detection transformers (DETRs) have gradually taken a dominant position in 2D detection thanks to their elegant framework. However, DETR-based detectors for 3D point clouds are still difficult to achieve satisfactory performance. We argue that the main challenges are twofold: 1) How to obtain the appropriate object queries is challenging due to the high sparsity and uneven distribution of point clouds; 2) How to implement an effective query interaction by exploiting the rich geometric structure of point clouds is not fully explored.To this end, we propose a simple and effective 3D DETR method (SEED) for detecting 3D objects from point clouds, which involves a dual query selection (DQS) module and a deformable grid attention (DGA) module. Extensive ablation studies on DQS and DGA demonstrate its effectiveness. Furthermore, our SEED achieves state-of-the-art detection performance on both the large-scale Waymo and nuScenes datasets, illustrating the superiority of our proposed method.
Authors:Zhe Liu,Jinghua Hou,Xiaoqing Ye,Tong Wang,Jingdong Wang,Xiang Bai
Affiliation: Huazhong University of Science and Technology, Baidu Inc.
Make Your ViT-based Multi-view 3D Detectors Faster via Token Compression
Slow inference speed is one of the most crucial concerns for deploying multi-view 3D detectors to tasks with high real-time requirements like autonomous driving. Although many sparse query-based methods have already attempted to improve the efficiency of 3D detectors, they neglect to consider the backbone, especially when using Vision Transformers (ViT) for better performance. To tackle this problem, we explore the efficient ViT backbones for multi-view 3D detection via token compression and propose a simple yet effective method called TokenCompression3D (ToC3D). By leveraging history object queries as foreground priors of high quality, modeling 3D motion information in them, and interacting them with image tokens through the attention mechanism, ToC3D can effectively determine the magnitude of information densities of image tokens and segment the salient foreground tokens. With the introduced dynamic router design, ToC3D can weigh more computing resources to important foreground tokens while compressing the information loss, leading to a more efficient ViT-based multi-view 3D detector. Extensive results on the large-scale nuScenes dataset show that our method can nearly maintain the performance of recent SOTA with up to 30% inference speedup, and the improvements are consistent after scaling up the ViT and input resolution.
Authors: Dingyuan Zhang, Dingkang Liang, Zichang Tan, Xiaoqing Ye, Cheng Zhang, Jingdong Wang, Xiang Bai
Affiliation: Huazhong University of Science and Technology, Baidu Inc.
WAS: Dataset and Methods for Artistic Text Segmentation
Accurate text segmentation results are crucial for text-related generative tasks, such as text image generation, text editing, text removal, and text style transfer. Recently, some scene text segmentation methods have made significant progress in segmenting regular text. However, these methods perform poorly in scenarios containing artistic text. Therefore, this paper focuses on the more challenging task of artistic text segmentation and constructs a real artistic text segmentation dataset. One challenge of the task is that the local stroke shapes of artistic text are changeable with diversity and complexity. We propose a decoder with the layer-wise momentum query to prevent the model from ignoring stroke regions of special shapes. Another challenge is the complexity of the global topological structure. We further design a skeleton-assisted head to guide the model to focus on the global structure. Additionally, to enhance the generalization performance of the text segmentation model, we propose a strategy for training data synthesis, based on the large multi-modal model and the diffusion model. Experimental results show that our proposed method and synthetic dataset can significantly enhance the performance of artistic text segmentation and achieve state-of-the-art results on other public datasets.
Authors: Xudong Xie, Yuzhe Li, Yang Liu, Zhifei Zhang, Zhaowen Wang, Wei Xiong, Xiang Bai
Affiliation: Huazhong University of Science and Technology, Adobe
PartGLEE: A Foundation Model for Recognizing and Parsing Any Objects
PartGLEE is a part-level foundation model for locating and identifying both objects and parts in images. Through a unified framework, PartGLEE accomplishes detection, segmentation, and grounding of instances at any granularity in the open world scenario. Specifically, we propose a Q-Former to construct the hierarchical relationship between objects and parts, parsing every object into corresponding semantic parts. By incorporating a large amount of object-level data, the hierarchical relationships can be extended, enabling PartGLEE to recognize a rich variety of parts. We conduct comprehensive studies to validate the effectiveness of our method, PartGLEE achieves the state-of-the-art performance across various part-level tasks and obtain competitive results on object-level tasks. These abilities enable PartGLEE to achieve deeper image understanding and allow multimodal large language models (mLLMs) to gain fine-grained understanding of images through prompts.
Authors: Junyi Li, Junfeng Wu, Weizhi Zhao, Song Bai, Xiang Bai
Affiliation: Huazhong University of Science and Technology, ByteDance Inc.
PSALM: Pixelwise SegmentAtion with Large Multi-Modal Model
PSALM is a powerful extension of the Large Multi-modal Model (LMM) to address the segmentation task challenges. To overcome the limitation of the LMM being limited to textual output, PSALM incorporates a mask decoder and a well-designed input schema to handle a variety of segmentation tasks. This schema includes images, task instructions, conditional prompts, and mask tokens, which enable the model to generate and classify segmentation masks effectively. The flexible design of PSALM supports joint training across multiple datasets and tasks, leading to improved performance and task generalization. PSALM achieves superior results on several benchmarks, such as RefCOCO/RefCOCO+/RefCOCOg, COCO Panoptic Segmentation, and COCO-Interactive, and further exhibits zero-shot capabilities on unseen tasks, such as open-vocabulary segmentation, generalized referring expression segmentation and video object segmentation. Open-sourced code is available at https://github.com/zamling/PSALM
Authors: Zheng Zhang, Yeyao Ma, Enming Zhang, Xiang Bai
Affiliation: Huazhong University of Science and Technology, Microsoft Research Asia
SC4D: Sparse-Controlled Video-to-4D Generation and Motion Transfer
Recent advances in 2D/3D generative models enable the generation of dynamic 3D objects from a single-view video. Existing approaches utilize score distillation sampling to form the dynamic scene as dynamic NeRF or dense 3D Gaussians. However, these methods struggle to strike a balance among reference view alignment, spatio-temporal consistency, and motion fidelity under single-view conditions due to the implicit nature of NeRF or the intricate dense Gaussian motion prediction. To address these issues, this paper proposes an efficient, sparse-controlled video-to-4D framework named SC4D, that decouples motion and appearance to achieve superior video-to-4D generation. Moreover, we introduce Adaptive Gaussian (AG) initialization and Gaussian Alignment (GA) loss to mitigate shape degeneration issue, ensuring the fidelity of the learned motion and shape. Comprehensive experimental results demonstrate that our method surpasses existing methods in both quality and efficiency. In addition, facilitated by the disentangled modeling of motion and appearance of SC4D, we devise a novel application that seamlessly transfers the learned motion onto a diverse array of 4D entities according to textual descriptions.
Authors: Zijie Wu, Chaohui Yu, Yanqin Jiang, Chenjie Cao, Fan Wang, Xiang Bai
Affiliation: Huazhong University of Science and Technology, DAMO Academy, Alibaba Group
Towards Dual Transparent Liquid Level Estimation in Biomedical Lab: Dataset, Methods and Practices
Accurately estimatesthe levels of such a liquid from arbitrary viewpoints is fundamental and crucial, especially in AI-guided autonomous biomedical laboratories for tasks like liquid dispensing, aspiration, and mixing. Existing methods focus only on single instances from fixed viewpoints, which significantly diverges from real-world applications.Hence, Prof.Xia Tian's team proposed a new benchmark for dual transparent liquid level height estimation, including a dataset, methods, andcode implementation. The proposed Dual Transparent Liquid Level Estimation Dataset (DTLD) contains 27,458 images covering four types of transparent biomedical laboratory vessels from multiple perspectives. Based on DTLD, the team proposed an end-to-end learning method to detect the liquid level contact line and estimate the liquid level.Aliquidlevelcorrection module was also introducedto enhance detection robustness. Experiments demonstrated that this method reduced the mean absolute percentage error of liquid level estimation by 43.4%.
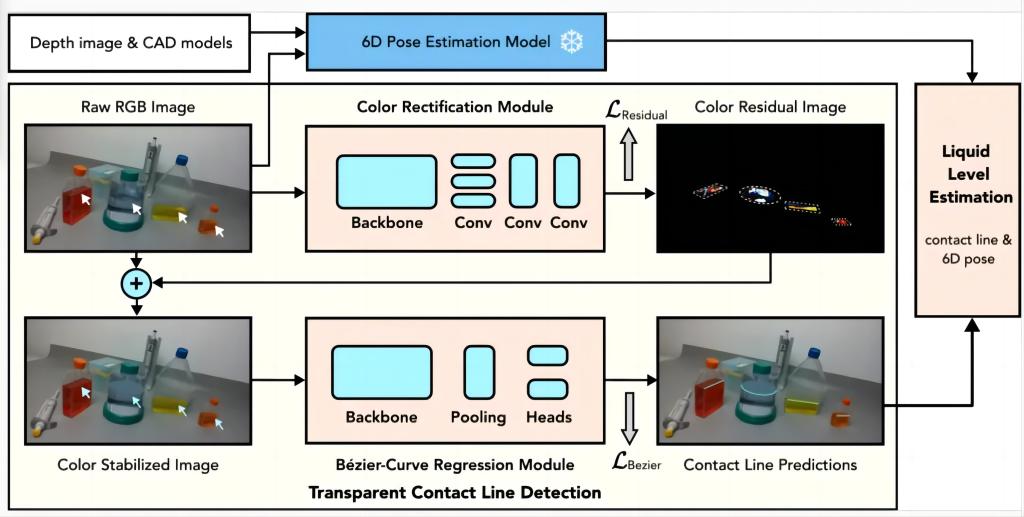
Authors:Xiayu Wang, Ke Ma, Ruiyun Zhong, Xinggang Wang, Yi Fang, Yang Xiao, Tian Xia
Affiliation: Huazhong University of Science and Technology,New York University Abu Dhabi
CoLA: Conditional Dropout and Language-driven Robust Dual-modal Salient Object Detection
The depth/thermal information is beneficial for detecting salient object with conventional RGB images. However, in dual-modal salient object detection (SOD) model, the robustness against noisy inputs and modality missing is crucial but rarely studied. To tackle this problem, we introduceaLanguage-driven Quality Assessment (LQA)tomitigate the impact of noisy inputs. Conditional Dropout (CD)is also introduced todealwith missing modalities. The CD serves as a plug-in training scheme that treats modality-missing as conditions, strengthening the overall robustness of various dual-modal SOD models. Extensive experiments demonstrate that the proposed method outperforms state-of-the-art dual-modal SOD models, under both modality-complete and modality-missing conditions.Thecodeof CoLAhas been open-sourced. Code implementation and morediscussionsare welcomed.
Authors:Shuang Hao,Chunlin Zhong,He Tang
Affiliation: Huazhong University of Science and Technology