The 2023 Computer Vision and Pattern Recognition Conference (CVPR’23) will be held in Vancouver, Canada from June 18 to June 22. The research paper “Detecting Backdoors During the Inference Stage Based on Corruption Robustness Consistency” guided byDr. Minghui Li has been accepted by CVPR’23. CVPR is an A-level international academic conference recommended by CCF and enjoysahigh academic reputation in the field of computer vision. This conference received a total of 9,155 submissions, and 2,360 papers were accepted, with an acceptance rate of about 25.78%.
Deep neural networks are proven to be vulnerable to backdoor attacks. Infected models will perform normal for clean inputs during the inference stage, but they will output incorrect predictions for inputs tampered by backdoor triggers. However, existing detection methods often require the defenders to have high accessibility to the victim model, extra clean data, or knowledge of the appearance of backdoor triggers, limiting their practicality. Thus, this paper proposes thetest-time corruption robustness consistency evaluation (TeCo), a novel test-time trigger sample detection method that only requires the hard label output of the victim model without any extra information. The journey begins with the intriguing observation that the backdoor-infected models have similar performance across different image corruptions for the clean images, but perform discrepantly for the trigger samples. Therefore,the TeCoisdesignedto evaluate test-time robustness consistency by calculating the deviation of severity that leads to prediction’s transition across different corruptions. Extensive experiments demonstrate that compared with state-of-art defenses during the inference stage, TeCo outperforms them on different backdoor attacks, datasets, and model architectures, enjoying a higher AUROC and longer stability time under different trigger types.
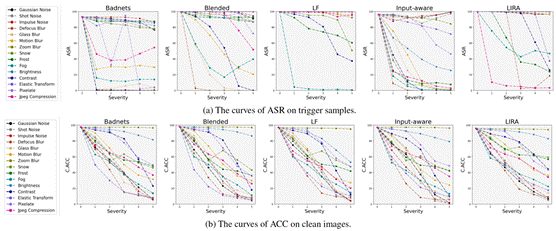
Figure 1 (a): The backdoor-infected model’s attack success rate (ASR) when trigger samples are tempered with different corruptions and levels of severity. (b): The accuracy (ACC) of clean images tempered with different corruptions and levels of severity. The curves separate loosely in (a), while the majority of curves gather more tightly in (b). This indicates that the backdoor-infected models have various corruption robustness against different image corruptions on trigger samples, but have similar robustness against different image corruptions on clean samples.
The 37th International Conference on Artificial Intelligence (AAAI Conference on Artificial Intelligence, AAAI’2023) was held in Washington, USA from February 7 to February 14, 2023. The research paper “PointCA: Evaluating the Robustness of 3D Point Cloud Completion Models Against Adversarial Examples” guided by Dr. Minghui Li has been accepted by AAAI’23. AAAI is an A-level international academic conference recommended by CCF and enjoys a high academic reputation in the field of artificial intelligence. This conference received a total of 8,777 submissions, and 1,721 papers were accepted, with an acceptance rate of about 19.6%.
This paper proposes PointCA, the first adversarial attack against 3D point cloud completion models. Point cloud completion, as the upstream procedure of 3D recognition and segmentation, has become an essential part of many tasks such as navigation and scene understanding. While various point cloud completion models have demonstrated their powerful capabilities, their robustness against adversarial attacks remains unknown. Existing attack approaches towards point cloud classifiers cannot be applied to the completion models due to different output forms and attack purposes. Therefore, this work proposes a new method to evaluate the robustness of point cloud completion models, namely PointCA adversarial attack. PointCA can generate adversarial point clouds that maintain highly similarity with the original ones while being completed as another object with totally different semantic information. Specifically, researchers minimize the representation discrepancy between the adversarial example and the target point set to jointly explore the adversarial point clouds in the geometry space and the feature space. Furthermore, to launch more covert attacks, researchers innovatively employ the neighborhood density information to tailor the perturbation constraint, leading to geometry-aware and distribution-adaptive modifications for each point.
Extensive experiments against different premier point cloud completion networks show that PointCA can cause a performance degradation from 77.9% to 16.7%, with the structure chamfer distance kept below 0.01. It is concluded that existing completion models are severely vulnerable to adversarial examples, and state-of-the-art defenses for point cloud classification will be partially invalid when applied to incomplete and uneven point cloud data.
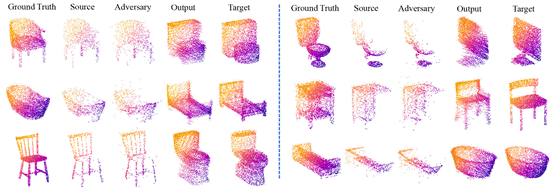
Figure 2: An illustration of targeted attack results. Source is the partial point cloud generated on Ground Truth from one view point. Tiny perturbations are added to Source to obtain the Adversary, whose completion Output is very close to the target.